What Is Cnn Algorithm?
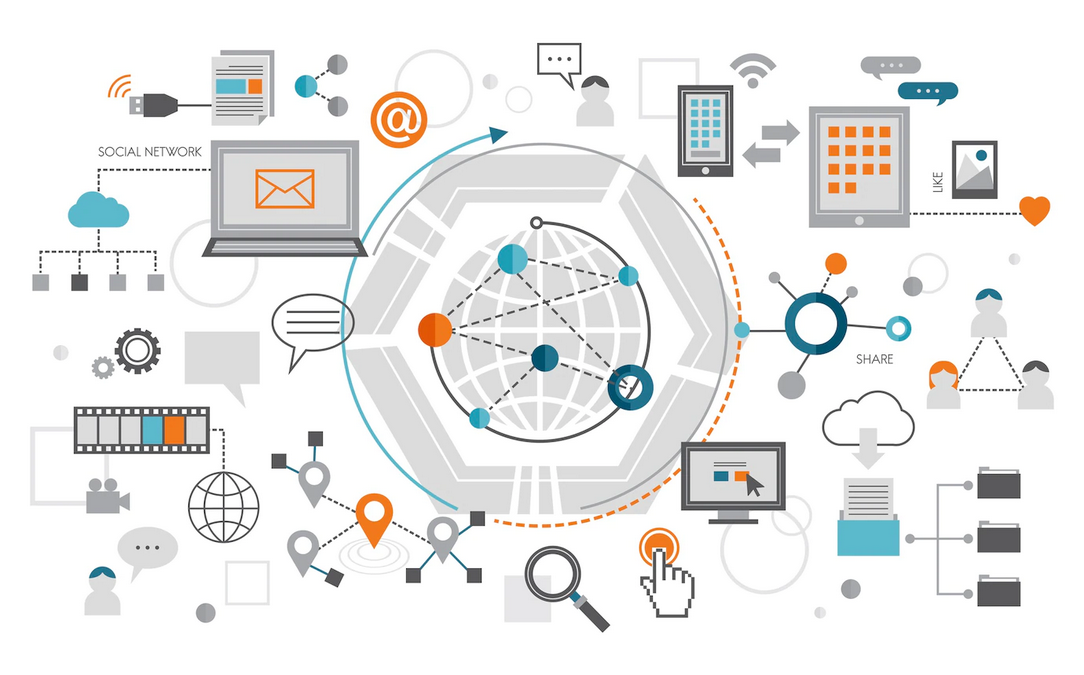
- The Role of CovNet for Feature Reduction
- ConvNet: A Pattern of Artificial Intelligence
- DropConnect: A Network Architecture for Data Mining
- Deep Learning for Image Processing
- Deep Learning in the Brain
- Convolution and non linear functions
- Training a Convolutional Neural Network
- The Softmax Function
- CNN: An Object Recognition System for Medical Image Processing
- Feature-based CNNs
- Anchor Boxes in a Discriminative Model
- CNN Classification: A Novel Approach
- Pattern Recognition by Neural Networks
- Perceptron: A Basic Neural Network
- Deep Learning and GANs
The Role of CovNet for Feature Reduction
The high priority features are important for prediction and high accuracy and the Role of CovNet is to reduce the image features so that it can be easily processed. There are two types of pool. Max Pooling gives the highest value from the portion of the image that is not covered by the filter.
Average Pooling gives the average of the values from the portion of the image that was used to make the filter. The system has to tell the weather if the input image belongs to the dataset or not. It is also called a problem.
ConvNet: A Pattern of Artificial Intelligence
Artificial Intelligence has been able to bridge the gap between the capabilities of humans and machines. Researchers and enthusiasts work on many aspects of the field to make amazing things happen. The domain of Computer Vision is one of the areas that is included.
The architecture of a ConvNet is similar to the pattern of the brain's visual cortex. Individual neurons only respond to stimuli in a restricted area of the visual field. A collection of fields overlap to cover the entire area.
A ConvNet can successfully capture the Spatial and Temporal dependencies in an image through the application of relevant filters. The architecture performs better in fitting the image dataset due to the reduction in parameters involved. The network can be trained to understand the image better.
DropConnect: A Network Architecture for Data Mining
In 1990 there was a report by Yamaguchi et al. The concept of max pooling is a fixed operation that calculates and distributes the maximum value of a region. They combined the two to realize a speaker independent isolated word recognition system.
They used a system of multiple TDNNs per word. The results of each TDNN were combined with max pooling and the outputs of the pooling layers were passed on to networks to perform word classification The full output volume of the convolution layer is formed by stacking the activation maps for all the filters along the depth dimensions.
Every entry in the output volume can be seen as an output of a neuron that looks at a small region in the input and shares parameters with the same activation map. It is impractical to connect all the cells in a previous volume because the network architecture doesn't take into account the spatial structure of the data. Convolutional networks exploit spatial correlation by using sparse local connections between the adjacent layers of the network.
A scheme to share parameters is used in the layers. It relies on the assumption that if a patch feature is useful to compute at a certain location, then it should be useful to compute at other locations. The depth slices are defined as a single 2-dimensional slice of depth.
CNNs are a form of non- linear down-sampling. Max pooling is the most common non- linear function to implement pooling. The maximum is output for each sub-region of the input image.
Deep Learning for Image Processing
Deep Learning has been a very powerful tool because of its ability to handle large amounts of data. The interest in using hidden layers has grown. Convolutional Neural Networks are one of the most popular deep neural networks.
The role of the ConvNet is to reduce the images into a form that is easier to process, without losing features that are critical for getting a good prediction. Neural networks are made of artificial neurons. Artificial neurons are mathematical functions that calculate the weighted sum of multiple inputs and outputs an activation value.
Each layer in a ConvNet creates several functions that are passed on to the next layer. CNNs provide in-depth results despite their power and resources. It is just recognizing patterns and details that are so small that they are noticed by the human eye.
Deep Learning in the Brain
CNNs are an example of deep learning, where a more sophisticated model pushes the evolution of artificial intelligence by offering systems that mimic different types of human brain activity.
Convolution and non linear functions
The first layer to be used to extract features from an image is convolution. Convolution uses small squares of input data to learn image features. It is a mathematical operation that takes two inputs.
Training a Convolutional Neural Network
There are a lot of different types of neural networks that can be used in machine learning projects. There are many different types of neural networks. The inputs to the nodes in a single layer will have a weight assigned to them that changes the effect that they have on the prediction result.
The weights are assigned on the links between the different nodes. It can take some time to tune a neural network. Neural network testing and training can be a balancing act between deciding what features are most important to your model.
A neural network with multiple layers is called a convolutional neural network. It processes data that has a grid-like arrangement. CNNs are great because you don't need to do a lot of pre-processing on images.
CNNs use a type of math called convolutions to handle the math behind the scenes. A convolution is used instead of matrix multiplication. Convolutions take two functions and return them to the original function.
CNNs apply filters to your data. CNNs are able to tune the filters as training happens, which makes them so special. That way the results are fine-tuned in real time, even when you have a lot of data.
The Softmax Function
CNN is a popular recognition method used in image processing. The structure of CNN includes two layers, one is the feature extraction layer, the other is the previous layer, and the input of each neuron is connected to the local receptive fields of the previous layer. The softmax function is a function that turns a K real value into a K real value that is 1
Many neural networks end in a final layer which outputs scores that are not easily scaled and which may be difficult to work with. The softmax function is used in neural network models to predict a multinomial probability distribution. Softmax is used as an activation function for multi-classification problems where class membership is required on more than two class labels.
1 answer. You are correct. The soft-max layer outputs a probability distribution.
CNN: An Object Recognition System for Medical Image Processing
Natural language processing and computer vision are combined in the form of an object recognition system called an object recognition system, or OCR. The image is recognized and then turned into characters. The characters are pulled together into a whole.
CNN is used for image tags and further descriptions of the image content. It is being used by the platforms for a more significant impact. Saving lives is a priority.
It is better to have foresight. You need to be prepared for anything when handling patient treatment. The method of creating new drugs is very convenient.
There is a lot of data to consider when developing a new drug. A similar approach can be used with the existing drugs. The most effective way of treating the disease was designed to be precision medicine.
Feature-based CNNs
A feature is a pattern obtained from the input data that aids in performing the desired image analysis. The CNN learns from the images. They emerge from the data frequently to get attention.
The fact that every human face has a pair of eyes will be used as a feature by the system to detect and learn from. The features of the objects are served by the edge contours. It refers to an operator that is applied to the entire image to transform the information in the image.
A smaller matrix is used in comparison to the input dimensions of the image, which include real valued entries. The name of the layer implies that it is fully connected with the previous layer. The last stages of CNN usually use fully- connected layers to connect to the output layer and build the desired number of outputs.
The final detail before implementing a CNN is to specify its design end to end and to decide on the layers of the Convolutional layers. The expression indicates multiple layers. The final layer is connected to the output layer.
Anchor Boxes in a Discriminative Model
The anchor boxes are used to predict the overlap of boxes. The network can predict more than one box at a time, but only if each prediction has a set of properties. The first prediction can be a rectangular box of a given form, while the second prediction can be a different form. The generative model aims at generating the most truthful output that will be fed into the discriminative model, which aims at differentiating the generated and
CNN Classification: A Novel Approach
CNN classification takes any input image and finds a pattern in the image, processes it, and categorizes it into various categories like car, animal, bottle, etc. CNN is used in learning to find similarities in images. The future of technology is being driven by a very complex and interesting algorithm.
Pattern Recognition by Neural Networks
Artificial intelligence has been trying to build computers that can make sense of visual data since the 1950s. The field of computer vision saw some improvements in the decades after. A group of researchers from the University of Toronto developed an artificial intelligence model that was more accurate than the best image recognition algorithms.
The developers use a test dataset to verify the accuracy of the CNN. The test dataset is not part of the training process. The output of the image is compared to the actual label of the image.
The test dataset is used to evaluate how good the neural network is at seeing and interpreting images. Despite their power and complexity, neural networks are pattern-recognition machines. They can use huge compute resources to find hidden and small visual patterns that might go unrecognized.
Perceptron: A Basic Neural Network
The input data is fed into the Neural Network system and then pre-processed by the layers of artificial neurons. The Input and Output layers are called Hidden Layers. Perceptron is a Neural Network that is basic in form.
It is a feed-forward artificial neural network. Each neuron is connected to another in the forward direction. Recurrent Neural networks can remember vital details such as the input they received, which makes them very precise in predicting what is coming next.
They are the most preferred method for sequential data like time series, speech, text, audio, video and many more. Recurrent Neural Networks can form a deeper understanding of a sequence and its context. The Recurrent Neural Networks have a memory that holds all the information.
Deep Learning and GANs
Deep learning is used in many industries to solve complex problems. Different types of neural networks are used to perform certain tasks. Artificial neural networks are used to perform computations on large amounts of data.
It is a type of machine learning that works on the structure and function of the human brain. Each of the nodes has information in the form of inputs. The inputs are calculated, and the bias added by the node.
The function of which neuron to fire is determined by the function of activation functions. CNN's are used for image processing and object detection. The first CNN was called LeNet.
It was used to recognize characters. LSTMs keep information over time. They are useful in time-series prediction because they remember inputs.
The four interacting layers of LSTMs communicate in a unique way. LSTMs are used for a lot of things, including time-series predictions. The LSTM can be used to input to the current phase and can be used to remember previous inputs.









X Cancel