What Is Cnn Geometry?
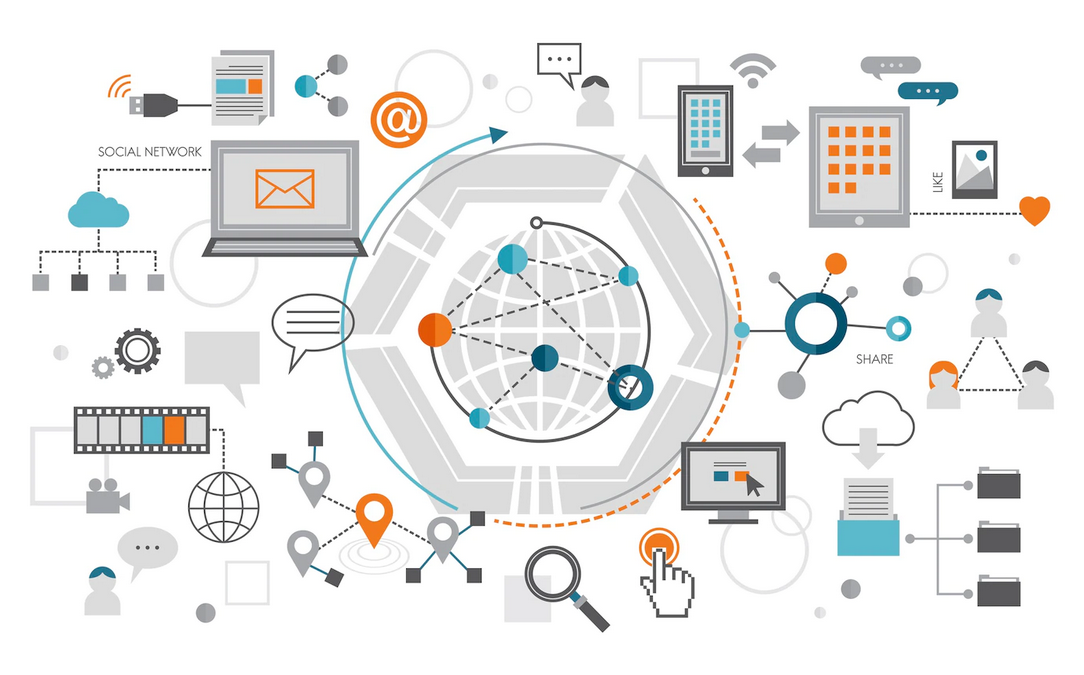
- Deep Learning in the Brain
- Geometricality in dimensions
- CNN: An Object Recognition System for Medical Image Processing
- LeNet: A Deeper Network
- Graph Theory and Deep Learning
- The final layer of the VGG19 network architecture
- A base of vectors for input layer
- The visual cortex
- On the input to pooling and FC layers
- Data Science Stack Exchange
Deep Learning in the Brain
CNNs are an example of deep learning, where a more sophisticated model pushes the evolution of artificial intelligence by offering systems that mimic different types of human brain activity.
Geometricality in dimensions
Geometricality in dimensions. Data is being used in training a neural network. Non-euclidean data is loyal to non-euclidean geometry.
There is a field of non-euclidean geometry that is not related to the EU. One of the most popular datatypes is an image. There is no idea of left, right, up or down on a graph.
CNN: An Object Recognition System for Medical Image Processing
Natural language processing and computer vision are combined in the form of an object recognition system called an object recognition system, or OCR. The image is recognized and then turned into characters. The characters are pulled together into a whole.
CNN is used for image tags and further descriptions of the image content. It is being used by the platforms for a more significant impact. Saving lives is a priority.
It is better to have foresight. You need to be prepared for anything when handling patient treatment. The method of creating new drugs is very convenient.
There is a lot of data to consider when developing a new drug. A similar approach can be used with the existing drugs. The most effective way of treating the disease was designed to be precision medicine.
LeNet: A Deeper Network
The network had a very similar architecture as LeNet but was deeper with more filters per layer and stacked layers. It included 11x11, 5x5,3x3 and a SGD with a momentum. Every layer had ReLU activations attached to it.
Graph Theory and Deep Learning
It is a strong title that shows that deep learning can be used on non-euclidean data. Non-euclidean data cannot fit in a two-dimensional space. A graph is a prime example of non-euclidean data.
A graph is a data structure which has entities connected to edges. A graph can be used to model a lot. You don't need an understanding of Graph Theory, you just need to read a bit about it so that you can use the software libraries that are required in the process.
You should have a clear idea of geometric deep learning for an excellent introduction to graph. If you already know the data you need to dispose of, then it is a best-case scenario for the data to be used to solve the problem. The input of the graph is input and the output of the graph is output with a certainty value.
It is the same as image classification, which has two main parts. The first part is the feature extractor which creates an optical representation of the input data. The fully connected layers are used to constrain the output regression.
The final layer of the VGG19 network architecture
The VGG19 architecture contained 16 layers of CNNs and three fully connected layers, the final layer is going to remain the same for all the network architectures.
A base of vectors for input layer
The filters in each layer can be used to create a basis of vectors that can be used to create a compact way to input the layer's input. The filters start incorporating more information from a larger area.
The visual cortex
The visual cortex has a small region of cells that are sensitive to visual fields. When some orientation edges are present, only some individual cells get fired inside the brain, and some respond when they are shown to horizontal or diagonal edges, which is nothing but the motivation behind Convolutional Neural.
On the input to pooling and FC layers
The input to the pooling layer is given and the input to the FC layer is given and the input to softmax is given.
Data Science Stack Exchange
Data science professionals, Machine Learning specialists, and those interested in learning more about the field can find answers on Data Science Stack Exchange. It takes a minute to sign up.









X Cancel